The Path to Rare Disease Clinical Trial Innovation
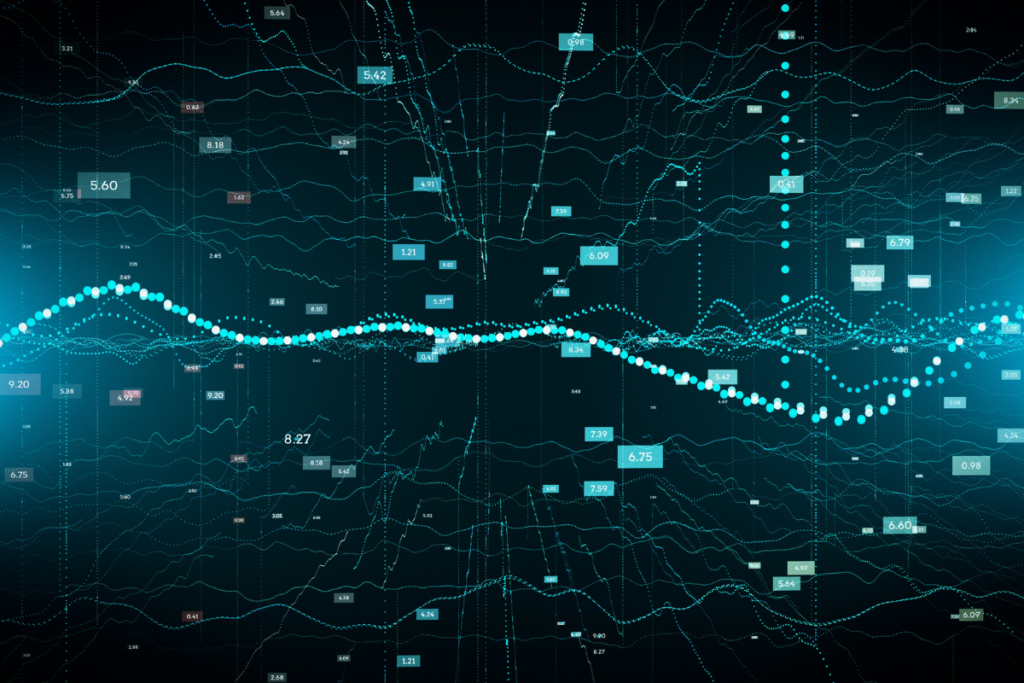
Executive Summary For decades, the pharmaceutical industry has faced the same recurring problems with clinical development: the struggle to fully recruit and retain enough patients, meet target timelines, and have trials conclude on time. Certainly, the industry does overestimate its ability to recruit, but a bigger issue is that study designs and protocol development seemingly fail to truly reflect patients’ lives, or account for the reality in the clinic. In fact, data shows the probability of success for any clinical development effort is 6.2% for orphan drug trials, compared with 13.8% overall, which translates to a 93.8% failure rate for orphan drug development efforts. Given the often progressive and irreversible nature of rare diseases, there is a need to increase efforts to find those undiagnosed patients, diagnose them earlier, and bring them into the frame when developing new treatment options. To achieve this, collectively as an industry, we must do more research into the rare disease patient population to characterise and better understand both the already diagnosed and the undiagnosed. We need this deeper understanding before deciding on the best clinical development strategy, finalising clinical trial design, and starting the enrolment of the patient population in a clinical study. To do that, clinical researchers and drug developers need to include much more knowledge and understanding of those people who are unknowingly living with the disease in the design of clinical development plans and study protocols. To find those people, there is a need to consult more extensively on the design of protocols, not just with the key opinion leaders, but also with physicians that are typically seeing and treating larger numbers of patients. One crucial factor with rare diseases is that the diagnostic journey is arduous and lengthy, often with many patients not being correctly diagnosed. As an example, a study found that 58% of Ehlers-Danlos syndrome (EDS) patients consulted more than five doctors, and 20% consulted more than 20[i]. So, when designing and recruiting for clinical trials, drug developers must first learn where the “as yet undiagnosed patients” are “hidden” – in other words, where they may be in the healthcare system, and which specialists they are seeing. It is those specialisms that need to be brought along in the diagnostic journey, so they can learn to identify rare disease patients within their practice. This is very well illustrated in the case of acute hepatic porphyria (AHP), where the view is that patients reside in the gastroenterology world, but, in fact, an even larger group is residing in other specialties. Another example is cited in Chapter 2. With novel approaches, such as the use of Machine Learning (ML), we can now highlight people who are not yet diagnosed as patients but are likely to be living with a disease, for their clinicians’ attention. Subtle indicators are derived from health care records by using ML, which would be difficult or nigh impossible for a doctor to recognise amidst the wealth of data already in front of them. Conducting thorough natural history studies of patients living with disease, but also including those wider populations of people suspected of living with disease but currently undiagnosed, can help to uncover sentinel events or detectable physiologic changes that are key predictors of disease progression or that are clinically important. These can provide an understanding of which subgroups of people living with the disease might benefit from a drug in development and should therefore be targeted for inclusion in the clinical trial. And, importantly, clinical researchers need to scrutinise the data and adopt insights gained by using ML models which will enable better clinical development strategy, design, and patient stratification. First, though, we need to understand the barriers and misconceptions about the art of the possible and address those directly. This paper explores the changing expectations of the regulators, the challenges the health industry continues to face, and the ways in which we can rethink the entire clinical development process – from development strategy to protocol design, to patient identification and recruitment – to achieve real breakthroughs in rare disease research and development. Chapter 2: Misconceptions and industry challenges The path to rare disease innovation begins with a better understanding of the complexity of each disease – a point well understood by the health authorities. As the US Federal Food and Drug Administration (FDA) has identified in its guidance on natural history studies, rare diseases can have substantial genotypic and/or phenotypic heterogeneity. As such, the natural history of each subtype, if it exists at all, may be poorly understood or inadequately characterised. Above all, a typical natural history study certainly does not include those people living with the disease that – in rare – often remain undiagnosed. There are two levels of undiagnosed patients: those who have had no diagnosis at all and have therefore not been matched with a disease, and those who have had a partial diagnosis but whose symptoms are not well characterised and therefore do not belong in a defined subgroup. As researchers learn more about rare diseases, they are starting to understand that different phenotypes may present with the involvement of different organ systems, with varying degrees of severity or rate of deterioration. As noted earlier, ML can help to elicit subtle indicators from electronic health records or claims data. However, during panel debates at recent orphan drug conferences, there seemed a strong bias towards the use of registries for research and patient characterisation, and there were clear misconceptions from both industry and regulators about the usability of primary care electronic medical records (or electronic claims data) for the purpose of early disease detection, be it in a traditional manner, or ML assisted. The limitations of registries While disease registries have a clear purpose, they are constrained by the fact that they tend only to contain data on patients that are known to have a given disease. By focusing only on rare disease data that already exists in patient registries, research and potential patient populations remain excluded from further studies. There are
Alpha-1 Antitrypsin Deficiency: Why millions remain undiagnosed
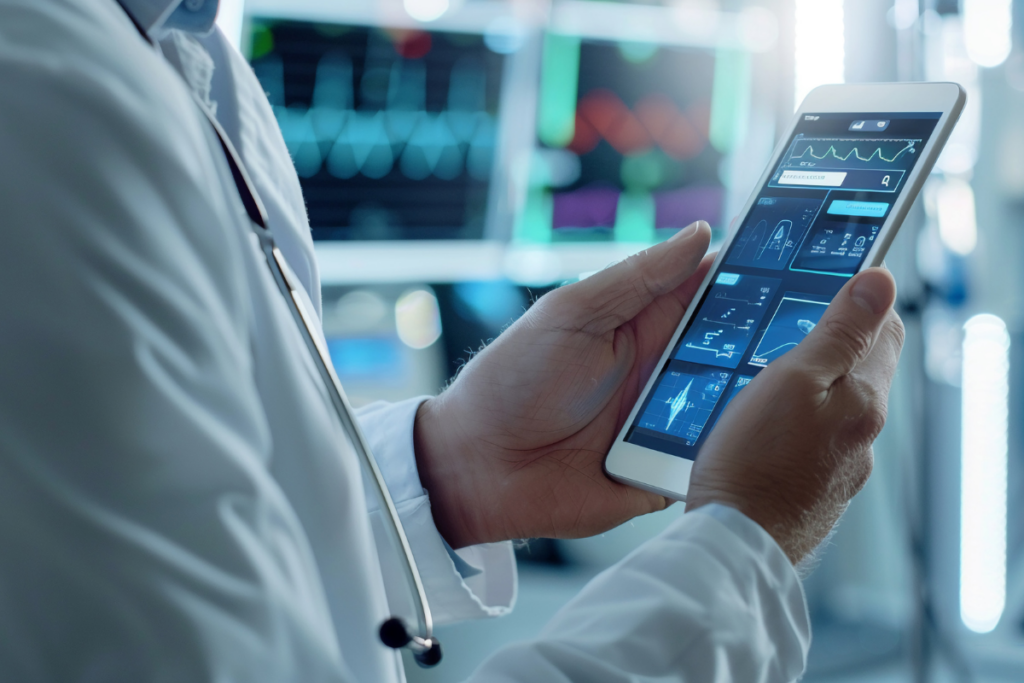
Alpha-1 antitrypsin deficiency (AATD), a rare genetic condition, can cause lung disease in adults with symptoms similar to chronic obstructive pulmonary diseases. AATD is largely underdiagnosed, with an estimated prevalence of 100,000 individuals with AATD in the United States (US); however, fewer than 10,000 individuals are diagnosed with the disorder. Previously, AATD was thought to affect only White individuals of European descent. Recent studies have shown that people of different races and ethnicities have genotypes consistent with those with moderate-to-severe AATD-related lung disease. We developed a prediction model to identify symptomatic patients of different races and ethnicities with likely risk of AATD using claims data from a large US database.